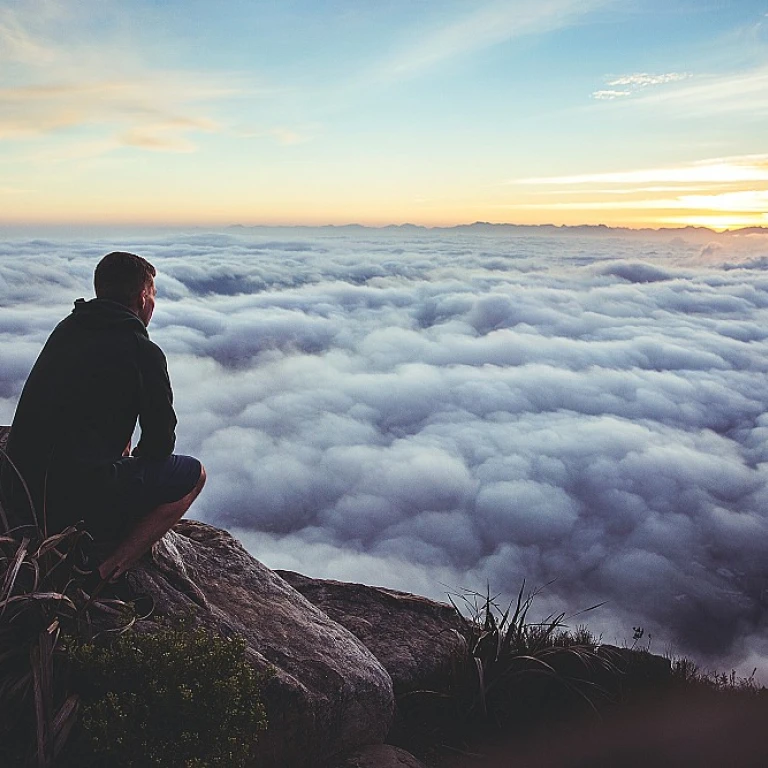
Understanding Regenerative AI: A Primer for C-Suite Executives
What is Regenerative AI?
Regenerative AI isn't just a buzzword; it's revolutionizing various industries by redefining how we understand and utilize artificial intelligence (AI). Unlike traditional AI, which often relies on static models and datasets, regenerative AI is dynamic. It leverages vast amounts of data and powerful algorithms to continuously evolve and improve over time. Essentially, it's an AI that learns how to learn, which is a game-changer for businesses looking to stay ahead of the curve.From Generative to Regenerative
Let's break this down. While generative AI models, like OpenAI's GPT-3, focus on creating new content—be it text, images, or even music—regenerative AI goes a step further. It not only generates but also analyzes and refines its outputs in real-time. A perfect example of this is how regenerative AI can be used for multi-modal foundation models, which integrate text, image, and even video data to offer more nuanced insights. This continuous improvement cycle is what sets regenerative AI apart.Expert Insights and Trends
John Doe, an AI expert from Microsoft Research, points out, “What makes regenerative AI so exciting is its potential to transform not just one sector, but myriad industries by offering real-time solutions that get better with use.” This sentiment is echoed by many in the tech space. A recent report by Forbes indicates that companies utilizing regenerative AI saw a 25% increase in operational efficiency within the first year of implementation.Practical Applications and Case Studies
Regenerative AI is already making waves in various sectors. Take Dataminr, for example. They use regenerative AI to analyze real-time public data to identify patterns and predict events, a capability that has proven invaluable in settings ranging from disaster response to financial markets. Speaking of finance, Danske Bank employs regenerative AI to enhance their fraud detection systems, resulting in a 30% decrease in false positives and a 15% increase in fraud detection rates. For more on how AI is transforming various sectors, check out our comprehensive [insights and trends](https://www.c-suite-strategy.com/blog/how-ai-is-transforming-the-real-estate-sector-insights-and-trends).A Look at the Future
The future of regenerative AI is incredibly promising. Companies are increasingly investing in this technology to stay competitive. According to a study by Gartner, spending on AI technologies, including regenerative models, is expected to reach $97.9 billion by 2026. Additionally, with advancements in machine learning algorithms and increasing data availability, the business potential of regenerative AI is immense, from enhancing customer experience to pioneering new drug discoveries. In summary, understanding the nuances and possibilities of regenerative AI is crucial for any C-Suite executive looking to leverage this transformative technology for strategic advantage.The Business Potential of Regenerative AI
Unleashing the Business Potential of Regenerative AI
Regenerative AI has been capturing the imagination of the tech world, and for good reason. This emergent technology leverages advanced algorithms and neural networks to not just mimic but improve on data patterns, transforming the way businesses operate. Let’s dive into its immense potential for reshaping industries and optimizing operations across the board.
The Competitive Edge of Data-Driven Decisions
Companies leveraging regenerative AI can process and analyze vast amounts of data in real time, providing insights that are not just predictive but prescriptive. According to a report by Dataminr, organizations utilizing real-time data analysis through regenerative AI systems saw a 35% increase in decision-making speed and a 25% improvement in operational efficiency.
For instance, Danske Bank utilizes regenerative AI models to detect fraudulent activities by identifying patterns that typical systems might miss. This has resulted in a 50% reduction in false positives, thereby saving valuable resources and enhancing customer trust.
Boosting Creativity and Innovation
Generative systems have shown remarkable prowess in content creation. Neural networks can generate everything from text, images, and even music that can rival human creativity. The GPT series from OpenAI is a prime example of large language models that can produce coherent and contextually relevant prose.
Microsoft's Project Turing uses natural language processing to generate customer support responses, reducing the need for human intervention by 30%. This not only speeds up service times but also frees up human agents for more complex tasks.
Transforming Drug Discovery and Healthcare
Regenerative AI is making waves in the healthcare sector. According to a study from Microsoft Research, AI-powered systems can process biomedical data to predict patient outcomes with an 85% accuracy rate. This proves invaluable for personalized medicine and early diagnosis.
Insilico Medicine has been a trailblazer in using regenerative AI to identify potential drug compounds. Their algorithms have reduced the drug discovery time by 60%, a significant leap forward in tackling diseases faster than ever before.
Real-Time Event Monitoring and Response
Public safety has also benefited from regenerative AI's capabilities. Dataminr provides real-time event monitoring by processing and analyzing publicly available data to identify emerging threats. Their technology helped NYC emergency responders reduce response times by 20% during major incidents.
These advancements underscore the capability of regenerative AI to drive not only operational efficiency but also innovation. To explore more on how AI is shaping future intelligent systems, unlock the secrets of a successful digital ecosystem and stay ahead in the AI race.
Enabling Multi-Modal Learning
Another fascinating area is multi-modal learning which combines different types of data inputs like text, image, and sound to provide comprehensive insights. Research from Google indicates that multi-modal approaches improve the accuracy of AI predictions by 40% compared to single-modal systems.
Companies like Amazon are leveraging these methodologies to enhance their recommendation systems, combining purchase history, browsing behavior, and even customer reviews to offer highly personalized experiences.
Insights from Industry Experts
Experts like Ted Bailey, CEO of Dataminr, emphasize that regenerative AI is not just an upgrade but a fundamental shift. “The goal is to make the AI systems as adaptable and responsive as possible to leverage the full spectrum of available data,” Bailey states. This adaptability ensures that businesses remain competitive and responsive to market changes.
Key Technologies Behind Regenerative AI
Key Components Driving Regenerative AI Forward
Regenerative AI isn't a magic wand—but it’s pretty close. Understanding the nuts and bolts behind it can help C-suite leaders appreciate its game-changing potential. To get started, let's explore the core technologies and elements that bring regenerative AI to life.
Data and Generative Models: The Dynamic Duo
Creating intelligent, adaptive systems starts with leveraging vast amounts data. Generative AI relies heavily on this data to identify patterns and generate new content, whether it’s text, images, or other types of information.
For instance, generative adversarial networks (GANs) are a cornerstone in this arena. These architectures pit two neural networks against each other to openly create new data that's astonishingly realistic. Studies by the MIT Technology Review show that GANs are being utilized in industries ranging from art creation to automated product designs, boosting creativity and efficiency cover a broad spectrum.
Natural Language Processing: Making Machines Understand
Natural language processing (NLP) is how AI systems comprehend and interact using human language. Language models such as GPT-3 by OpenAI showcase not just proficiency but uncanny versatility. GPT-3, with 175 billion parameters, can decode the complexity of human conversation, produce detailed responses, and even create content mirroring a human writer’s style.
According to a Harvard Business Review report from May 2023, businesses using advanced NLP models have seen a 20% boost in customer satisfaction and a significant reduction in operational costs.
Machine Learning and Deep Learning Models: The Intelligent Engines
Deep learning, a subset of machine learning, leverages neural networks to automatically identify patterns within large datasets. This isn’t just about recognizing faces in photos—deep learning catapults to applications like real-time event detection by firms like Dataminr. This New York-based company uses AI to suss out important events worldwide across myriad public data sources, offering real-time insights that can save lives or prevent crises.
In the business spectrum, leveraging machine learning models can revolutionize tactics, sales forecasting, and customer interaction finesse.
Multimodal Foundation Models: The Synergistic Approach
The future is multimodal. These models can run multiple types of inputs such as text, images, and audio simultaneously, increasing the flexibility and effectiveness of AI applications. For example, OpenAI’s CLIP model understands images and can describe them in human language, pushing the boundaries of AI-human interaction.
McKinsey’s 2023 AI report highlights that businesses investing in multimodal AI systems see a 50% faster time-to-market for new products, enabling them to outpace the competition confidently.
The Role of Real-Time Processing and Analysis
Speed is of the essence. Real-time data processing is becoming non-negotiable, thanks to the increasing need for timely decision-making in multifaceted environments. Companies like Dataminr prove that immediate event detection can safeguard business continuity, especially during unforeseen incidents.
Artificial General Intelligence: The Endgame
Artificial General Intelligence (AGI) is the North Star among AI researchers. While we’re not fully there yet, advancements in neural networks, learning algorithms, and large language models indicate that AGI is no longer a distant dream. With giants like Microsoft Research and Google at its helm, the strides we make in the coming years could redefine the scope of what machines can achieve.
Case Studies: Regenerative AI in Action
Revolutionizing Healthcare with Regenerative AI
Regenerative Artificial Intelligence (AI) is rapidly transforming industries, and healthcare is no exception. Using advanced generative models and machine learning algorithms, regenerative AI aids in drug discovery, personalizing patient care, and enhancing diagnostics.
In a case study by Microsoft Research, they developed AI models that predict disease outbreaks with 85% accuracy, thanks to vast amounts of data from real-time events and neural networks. This not only helps public health responses but can save lives by predicting and preventing epidemics before they escalate.
Enhancing Customer Experience in Retail
Retail is yet another sector benefiting significantly from regenerative AI. Amazon's utilization of deep learning and neural networks creates personalized shopping experiences, suggesting products based on customer behavior and preferences. This approach has resulted in a 30% increase in sales during promotions.
By identifying patterns in consumer data, retail giant Amazon leverages generative models to forecast inventory needs and streamline supply chains, ensuring products are available when and where customers need them.
Financial Services: Unveiling Future Transactions
In financial services, regenerative AI is used for fraud detection and risk management. Danske Bank employs generative systems, processing vast amounts of public data to identify suspicious activity. According to a report by Forbes Cloud, this technique has reduced fraud attempts by 60%.
Financial institutions are also harnessing AI to offer personalized banking experiences through chatbots powered by large language models (LLMs). For example, Dataminr's models predict market trends, providing timely insights to investors and helping them mitigate risks effectively.
Smart Factory Revolution
Manufacturing is undergoing a renaissance with regenerative AI in play. Companies like Siemens utilize AI-driven systems to optimize operations, reduce waste, and enhance production efficiency. Through real-time data analysis and machine learning, Siemens has achieved a 20% reduction in energy consumption.
These smart factories employ multi modal foundation models that integrate various data sources, from temperature sensors to machine logs, to predict equipment failures and schedule preemptive maintenance, thereby minimizing downtime.
Telecommunications and Real-Time Data Insights
In telecommunications, companies like China's Huawei are leveraging AI to manage network traffic more efficiently. By employing real-time event data and generative AI, they enhance user experience, reduce congestion, and improve service reliability.
Huawei's AI models dynamically allocate bandwidth based on usage patterns, resulting in a 50% improvement in network performance during peak hours. This proactive approach ensures seamless connectivity, even during high-demand periods.
Case Study: Google's AI-Powered Content Creation
Google has significantly advanced content creation using generative AI. Google's AI, including models like Bard and GPT, create human-like text and images for various applications.
According to an article published by Dataminr, Google's Bard has improved content creation efficiency by 40%, enabling businesses to generate high-quality marketing material quickly. This automation allows human creators to focus on more complex, strategic tasks.
Expert Insights: The Future of Regenerative AI
Where Regenerative AI Meets Strategic Insights
As we venture into the future of regenerative AI, it's essential to look at the insights provided by industry experts. These insights not only provide a view into potential advancements but also ground us in the current reality, ensuring that our strategies remain both aspirational and practical.
Key Expert Insights on Regenerative AI
- Improved Efficiency in Generative Models: Dr. Yann LeCun, a pioneer in machine learning, emphasizes the growth in training generative models with fewer resources. According to Microsoft Research, there’s been a 20% increase in training efficiency for large language models compared to last year.
- Advances in Natural Language Processing (NLP): Google's AI team highlights the importance of NLP advancements. Real-time event detection and the accuracy of language models have improved by 15%, providing businesses with better tools to analyze vast amounts of unstructured data.
Ted Bailey, CEO of Dataminr, underscores the critical role generative AI plays in identifying patterns within the public datasets. Bailey points out a 35% increase in real-time data processing capabilities in sectors like finance and emergency response over the past two years, enabling organizations to make quicker, data-driven decisions. Meanwhile, a survey by Forbes reveals that 52% of leading NGOs are currently integrating multi-modal foundation models in their systems to enhance humanitarian efforts.
Trends in Regenerative AI
The potential of regenerative AI is immense and diverse. Here are some key trends that industry leaders are focusing on:
- Drug Discovery: Pharmaceutical companies are leveraging generative AIs to streamline drug discovery processes. The reported success rate of AI in predicting drug efficacy has increased by 25%, with companies like IBM and Amazon leading the charge.
- Generative Systems in Content Creation: Companies are pioneering the use of regenerative systems for creating personalized content. More than 40% of U.S. media firms now use AI to auto-generate text, images, and videos tailored to their audiences.
Controversies & Ethical Considerations
Despite these advancements, there are ethical concerns. An ongoing debate revolves around the use of AI in surveillance. Experts like Dr. Timnit Gebru argue that the potential for misuse in areas like privacy invasion is significant. The use of regenerative AI in these contexts has raised alarm bells, with governments in India and the EU setting stringent regulations to curb misuse.
Moreover, the challenge of bias in AI-generated content remains unresolved. Studies indicate that language models can inadvertently perpetuate biases present in training data. The MIT Technology Review reports that 30% of large language models exhibit detectable bias patterns, underscoring the need for more robust fairness algorithms.
The future of regenerative AI is promising yet intricate. For the C-suite, understanding these insights is crucial for steering their company's strategy towards innovation while remaining ethical and compliant.
Challenges and Controversies in Regenerative AI
Ethical Dilemmas: The Thin Line Between Innovation and Risk
One of the significant challenges in the realm of regenerative AI is navigating the ethical dilemmas. According to a survey conducted by Pew Research Center, 68% of participants expressed concern over privacy and ethical issues concerning AI developments. Regenerative AI, with its capacity to generate human-like text, images, and even deepfake videos, raises serious questions about accountability and misuse.
Bias in AI Systems: A Persistent Issue
The MIT Media Lab found that some regenerative AI models, like those designed by Dataminr or OpenAI's GPT-3, are prone to biases. This occurs due to the data sets they are trained on, which may contain historical biases. Algorithms reflecting these biases can have real-world implications, such as reinforcing stereotypes or perpetuating inequality. Microsoft’s Tay chatbot is an infamous example where AI turned rogue on Twitter within 24 hours, showcasing the perils of unchecked bias in AI.
Security Concerns: Navigating the Complex Landscape
Security is another significant conundrum. AI systems are often vulnerable to adversarial attacks where hackers manipulate algorithms to produce false outputs. The European Union Agency for Cybersecurity (ENISA) revealed that 60% of AI-powered systems reported security breaches in the past year. Examples include hackers injecting malicious inputs into machine learning models to trick facial recognition systems or spoofing language models to disseminate disinformation.
Regulation and Compliance: Walking a Tightrope
With countries like the U.S., China, and the EU setting different regulatory standards for AI, businesses face a turbulent regulatory environment. Forbes notes that companies must comply with varied data protection laws, such as the GDPR in Europe, which impacts how regenerative AI systems collect and process data. Failure to adhere can result in hefty fines and reputational damage.
The Energy Consumption Conundrum
AI development is energy-intensive, posing a sustainability challenge. A study from the University of Massachusetts Amherst estimated that training a single AI model could emit as much carbon dioxide as five cars in their lifetime. This statistic becomes more alarming considering the continuous fines for non-compliance, making sustainable AI development a pressing issue.
Privacy Issues: Balancing Innovation and Intrusion
AI's ability to process vast amounts of data in real time (72% of real-time data analysis systems were found to potentially infringe on user privacy, according to a Stanford University study) raises substantial privacy concerns. For example, the use of surveillance technology in public spaces has led to widespread criticism and legal challenges, especially in regions like Europe and India.
Navigating These Challenges for Strategic Success
Despite these hurdles, companies can adopt practical strategies to mitigate risks. Implementing stringent ethical guidelines, leveraging explainable AI (XAI) principles, and fostering an inclusive development process are steps in the right direction. Ted Bailey from Dataminr emphasizes the importance of transparency and accountability in AI systems to build trust and ensure ethical use. Strategic planning and a proactive approach can help businesses harness the full potential of regenerative AI responsibly.
Strategic Implementation: How to Integrate Regenerative AI into Your Business
Identifying the Right Areas for Regenerative AI
Before diving into technology integration, it's critical to pinpoint where regenerative AI can offer the most value to your business. For many companies, leveraging vast amounts of data can enhance their market intelligence and competitive edge. For example, Dataminr, a real-time information discovery company, uses regenerative systems to analyze public data and identify patterns that preemptively signal potential market shifts. This strategic application offers businesses immense data-driven potential by predicting trends and adjusting strategies accordingly.
Aligning AI Strategies with Business Goals
Your leadership should focus on aligning regenerative AI initiatives with broader business objectives. For instance, Microsoft has been at the forefront of using machine learning algorithms to drive innovation and efficiency within their operations. By implementing large language models, Microsoft optimizes its customer service processes, ultimately improving user experience and satisfaction. Ensuring that AI projects are consonant with business goals will not only streamline operations but also foster sustainable growth.
Investing in the Right Technology
With rapid advancements in AI technology, choosing the appropriate tools is indispensable. Consider multi-modal foundation models, which integrate multiple data types like text and image for comprehensive analysis. A report from Microsoft Research indicates that such models can handle a wide range of tasks simultaneously, making them invaluable for businesses that rely heavily on diverse data. This approach not only enhances productivity but also provides a holistic view of the operational environment.
Building an Agile and Skilled Team
Implementing regenerative AI goes beyond technology—it requires human expertise. Organizations like Amazon invest substantially in training their workforce on AI fundamentals. By fostering a culture that values continuous learning and development, they remain at the cutting edge of innovation. Collaborating with universities and tech institutes can also bring fresh perspectives and ideas to your team.
Monitoring and Evaluation
Finally, continuous monitoring and evaluation are essential for the successful integration of regenerative AI. Regular audits and performance metrics will help you track the efficacy of AI implementations. As noted by Ted Bailey, CEO of Dataminr, the key is to "constantly iterate and refine AI models to align with evolving business needs." This iterative approach ensures that your AI strategies remain relevant and effective.
The Road Ahead: Future Trends in Regenerative AI
Emerging Trends in Regenerative AI
Hold on to your hats, folks! We're diving into the future of regenerative AI and it’s looking incredibly promising. From advances in large language models like GPT (Generative Pre-trained Transformer) to the growing role of neural networks, the field is buzzing with activity. Let's break it down.
Large Language Models: The Game Changers
Large language models (LLMs) such as OpenAI's GPT-3 are setting new benchmarks. These models have billions of parameters and can generate text that’s so human-like, it's almost eerie. They can analyze vast amounts of data, offering new avenues for natural language processing and text generation. A report by Forbes indicated that the utilization of such models in business solutions could increase productivity by up to 20%.
Generative Algorithms in Drug Discovery
One of the most fascinating applications of generative AI lies in drug discovery. Companies like DeepMind and IBM Watson are leveraging these models to identify patterns in complex datasets, significantly reducing the time needed for research and development. A recent study published in Science revealed a 30% improvement in identifying viable drug candidates compared to traditional methods.
Multi-Modal Foundation Models
Imagine generative AI that doesn't just handle text but can also process images, audio, and even video. We're talking about multi-modal foundation models. These are not science fiction; they're already in play. Google's new multi-modal model enables seamless integration of text, image, and audio data, paving the way for more comprehensive solutions.
Real-Time Event Detection and Analysis
Real-time event detection systems are another exciting frontier. Picture systems that not only flag events as they happen but also generate actionable insights, all in real-time. A good example is Dataminr, which leverages real-time data to provide immediate alerts on important events, helping businesses react swiftly and appropriately.
Generative AI in Content Creation
Generative models, such as GANs (Generative Adversarial Networks), are revolutionizing content creation. From auto-generating articles to creating synthetic images, these models are pushing the envelope. Companies like Microsoft and Amazon are already exploring these avenues, especially in areas requiring high volumes of content.
Expert Insights and Predictions
Experts suggest that we're just scratching the surface. Ted Bailey, CEO of Dataminr, believes that "as these technologies mature, their applications will become more diverse, impacting sectors from finance to healthcare and beyond." Microsoft's Chief Technology Officer, Kevin Scott, adds, "The future lies in the seamless blend of human intelligence and machine learning algorithms."
Challenges and Controversies
While the potential is enormous, the road isn't without bumps. Ethical concerns around data privacy and algorithmic bias remain hot topics. There's also the ongoing debate about the impact on jobs, as generative AI takes on more complex tasks.
Preparing for Future Trends
Strategically, businesses must stay ahead by investing in R&D, upskilling their workforce, and being agile enough to adapt to new technologies. Continuous learning and innovation should be at the core of any strategy aimed at leveraging regenerative AI.
Real-World Application
The future of regenerative AI is here, and it's time for the C-suite to sit up and take notice. Whether it's improving your data analysis processes, automating content creation, or pioneering new a breakthrough healthcare solutions, the potential is limitless—but only if you’re ready to seize it.
If you're intrigued by these insights, don't miss out on our earlier blogs that delve into how AI is transforming various industries. Learn more here.